AI Accurately Detects LV Dysfunction Using Single-Lead Apple Watch ECG
Though work remains before this approach is broadly adopted, experts see potential for use as a screening tool.
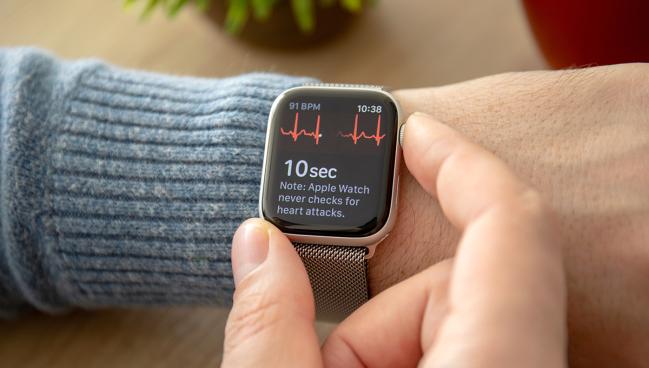
An artificial intelligence (AI) algorithm trained to interpret single-lead ECGs from the Apple Watch accurately detected signs of subclinical left ventricular systolic dysfunction in a proof-of-concept study, opening up the possibility of using measurements obtained at home to find and treat patients before they progress to more-severe disease.
With an area under the receiver operating characteristic curve (AUC) of 0.89, the AI-ECG algorithm has a performance that compares favorably with other screening tests commonly used in medicine, like mammography for breast cancer and cervical cytology for cervical cancer, researchers led by Zachi Attia, PhD (Mayo Clinic, Rochester, MN), noted in a study published online this week in Nature Medicine. Some of the results were presented earlier this year at Heart Rhythm 2022.
Senior author Paul Friedman, MD (Mayo Clinic), told TCTMD this is a “proof of concept” for using the AI algorithm to interpret single-lead ECGs from a smartwatch, adding that further validation and implementation work is necessary before rolling out the approach more broadly.
Implementation tests at other hospitals are expected to begin over the next couple of months, he said. “We have demonstration projects that look incredibly exciting and encouraging, but we need to continue—as with any new tool—to vet, validate, and prove that in the real world it improves human lives, lowers the risk of death, and lowers the risk of morbidity. And that is going to require more study.”
Other experts echoed both the call for additional research and the positive outlook for the approach.
Rhodri Davies, MBBS, PhD (University College London, England), said the study was “very promising,” touting as strengths the prospective design and the use of data separate from those used to derive the algorithm initially.
If the algorithm is validated in future studies and implementation issues are worked out, the approach could find a place in clinical practice, he indicated. “It shouldn’t replace echocardiography or other means of measuring LV ejection fraction properly, but as a screening tool it’s potentially really useful.”
Taking the Next Step
Multiple research groups have developed AI algorithms, mostly applied to 12-lead ECGs obtained in clinical settings, to detect a variety of conditions, including valvular heart disease, hypertrophic cardiomyopathy, amyloid heart disease, electrolyte abnormalities, and silent arrhythmias. The Mayo Clinic team previously showed that an algorithm trained to detect signals on 12-lead ECGs increased detection of LV systolic dysfunction by more than 30% compared with usual care, when deployed across primary care practices in the EAGLE study.
But, until now, the approach had not been shown to work when the algorithm was adapted to interpret single-lead ECGs, such as those obtained with the Apple Watch.
It shouldn’t replace echocardiography or other means of measuring LV ejection fraction properly, but as a screening tool it’s potentially really useful. Rhodri Davies
For the current study, the investigators emailed patients who had previously been treated at Mayo Clinic and had downloaded the mobile patient app to invite them to participate. Those who accepted were asked to download the Mayo Clinic ECG study app, which would send ECGs measured on the Apple Watch to a secure dashboard paired with the patient’s electronic medical record. Friedman explained that the Apple Watch was chosen because the company had made all of the raw ECG data available through the Apple Health Kit; the company was not involved in the study.
Overall, 3,884 patients were enrolled, of whom 2,454 (63%) sent at least one ECG during the study period. In this latter group, pulled from 46 US states and 11 countries, mean age was 53, 56% were women, and 88% were white. A total of 125,610 ECGs were sent to the secure dashboard, with 92% of patients using the ECG app more than once and half using it more than five times. Most of the ECGs (78.5%) were classified as normal sinus rhythm by the Apple Watch, 5.1% as atrial fibrillation, and 16.4% inconclusive.
To assess performance in identifying subclinical cardiac dysfunction, the researchers focused on 421 patients who sent at least one ECG within 30 days of a clinically indicated echocardiogram. Of those, 16 (3.8%) had an ejection fraction of 40% or less, with most of that group having minimal or no symptoms of LV systolic dysfunction.
Friedman said the AI-ECG algorithm “worked very well” for the detection of cardiac dysfunction, noting that the AUC of 0.89 is similar to what is seen with a treadmill test (AUC 0.85). “An ECG recorded from a consumer device, when we apply this AI analysis to it, can detect what could potentially be a life-threatening silent heart condition,” he said.
Potential Uses
Friedman called this study a first test of this approach, one that needs to be followed by additional research. But if the findings are validated, there are a couple of potential roles for the technology in clinical practice. First, it could be used to screen for subclinical LV dysfunction in high-risk individuals, such as those who are older or who have diabetes, and then initiate appropriate treatments when it’s detected.
Another possibility is for patients undergoing chemotherapy for cancer, who could monitor for treatment-related cardiac damage using ECGs obtained on a smartwatch rather than going in for periodic echocardiograms, he said.
Partho Sengupta, MD (Robert Wood Johnson University Hospital, Rutgers Robert Wood Johnson Medical School, New Brunswick, NJ), said demonstration of the accuracy of the AI-ECG approach for detecting cardiac dysfunction with single-lead ECGs—with performance similar to when the algorithm was applied to 12-lead ECGs—is a “pretty spectacular development” because it brings the approach into people’s homes.
He raised some questions about the size of the study, the relative lack of racial/ethnic diversity among the participants, and whether all patients were truly asymptomatic, and he stressed the need for additional research conducted in other centers and countries. With those caveats, he called this “a fantastic technological innovation. . . . It democratizes the ability to get that information.”
This is extremely promising. Partho Sengupta
Sengupta also saw a role for this approach as a screening tool if the results of the study are borne out in future research. Patients with subclinical LV dysfunction either could be treated early, preventing progression to symptomatic heart failure, or examined more closely to uncover the reason for the reduced ejection fraction, which could then be treated, he suggested.
He stressed that any potential impact on clinical outcomes remains to be proven in randomized trials. Future studies also should assess whether all patients with heart failure—regardless of ejection fraction—can be identified using the AI-ECG algorithm, he said.
Implementation Challenges
There are challenges when it comes to implementing such an approach more widely in clinical practice, the experts agreed. Davies pointed to the need for the infrastructure required to collect the ECG data and for the right clinician to be checking the information and acting on it. There’s no point in alerting a patient to the fact that their watch picked up signs of LV dysfunction if there isn’t a connection to a cardiologist or other physician who can take a history and perform the necessary imaging tests to confirm the finding, he said.
Also, as something like this is introduced, “you’ve got to be careful about who you apply it to,” Davies said, noting the algorithm would be expected to perform better in older, higher-risk patients than in younger, healthier individuals. False-positives need to be minimized as well, he noted.
Friedman agreed that there is a risk of false-positives if screening is applied to the wrong types of individuals, stating that there is a need for some operational processes to ensure that it’s being offered to appropriate populations.
The technical aspect of screening is already built in, as the secure dashboard has already been built, Friedman said, adding that even though most hospitals don’t have a similar platform yet, it’s likely more will get them in the next couple of years.
When optimally integrated into a health system’s electronic medical record system, this strategy should not add a lot of extra time for a clinician, especially as hospitals gain experience with it, Friedman stressed. “Once it’s in that situation, I don’t think it will significantly impact workflows. It has to be seamless for it to be effective. Doctors are swamped. We can’t make them busier.”
Another question around implementation, Sengupta pointed out, is whether individuals will be subject to “alarm fatigue” if they’re constantly getting alerts about potential problems from their smartwatches. And that is one aspect that needs to be addressed by studying AI-ECG screening and its impact on clinical outcomes in randomized trials.
Despite the lingering questions, Sengupta said he is enthusiastic about the possibilities, particularly if AI-ECG is combined with other types of wearable monitors in the future.
“This is extremely promising,” he said. “This is an important technological step that has been achieved.”
Todd Neale is the Associate News Editor for TCTMD and a Senior Medical Journalist. He got his start in journalism at …
Read Full BioSources
Attia ZI, Harmon DM, Dugan J, et al. Prospective evaluation of smartwatch-enabled detection of left ventricular dysfunction. Nat Med. 2022;Epub ahead of print.
Disclosures
- The publication was supported by the Ted and Loretta Rogers Cardiovascular Career Development Award Honoring Hugh C. Smith, MD. The Mayo Clinic Center for Digital health funded and developed the iPhone app used in this study. The ECG dashboard used for clinician review was developed and supported by the Department of Cardiovascular Medicine. No technical or financial support was received from Apple.
- The AI ECG algorithm to detect LV dysfunction was licensed by Mayo Clinic to Anumana, Eko health. Attia, Friedman, and several others may benefit financially from commercialization of the algorithm.
- Sengupta reports being an advisor to RCE Technologies and Echo IQ.
- Davies reports no relevant conflicts of interest.
Comments