Machine Learning Based on ECG, Clinical Data Predicts Coronary Calcium
If validated in future studies, these techniques can be used to enhance physicians’ clinical judgment, one researcher says.
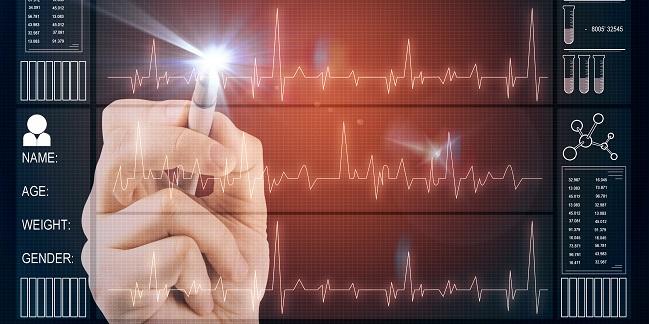
Machine-learning models that incorporate common clinical features along with ECG findings may help identify patients who are likely to have coronary artery calcium (CAC), with potential implications for further testing and communication of risks to patients, a pilot study indicates.
Moreover, in a cohort of patients undergoing invasive coronary angiography, the model designed to predict the presence of high levels of calcium was able to identify patients who had increased risks of significant coronary stenosis, MACE, and revascularization over 2 years of follow-up.
“Machine-learning techniques can extract information from electrocardiographic data and clinical variables to predict CAC score categories and similarly risk-stratify patients with suspected coronary artery disease,” the investigators conclude.
Senior author Partho Sengupta, MD, MBBS (West Virginia University Heart and Vascular Institute, Morgantown), noted that even though CT-based CAC scoring is an established method for stratifying patient risk, it has limitations—it may not be available in all parts of the world and there may be concerns about radiation exposure. In some instances, too, there may be pushback from payers regarding reimbursement.
That motivated the researchers to look for other ways to predict whether a patient might have coronary calcium, which could cut down on the number of CAC scans that would ultimately have to be performed.
It never is going to replace you. It’s only going to make you better and allow you to have your clinical judgment made more accurately. Partho Sengupta
In this study, with lead authors Peter Farjo, MD, and Naveena Yanamala, PhD (West Virginia University Heart and Vascular Institute), the investigators used machine-learning approaches that incorporated clinical features and ECG findings to develop models to predict CAC score categories—specifically, Agatston scores of 0 or ≥ 400.
This was done as a post hoc substudy of a prospective, multicenter trial designed to develop a machine-learning model from surface ECGs to predict the presence of diastolic dysfunction. The current analysis included 534 patients (mean age 58 years; 43% women) who underwent both CAC scoring and a 12-lead signal-processed surface ECG in the same visit.
For the model designed to predict no coronary calcium (Agatston score of 0), ECG variables were not included because they did not improve performance over the clinical features alone. This model performed had a sensitivity of 92%, specificity of 70%, and accuracy of 75%.
The model designed to predict an Agatston score of 400 or more included both clinical and ECG features. It also performed well, with a sensitivity of 91%, specificity of 75%, and accuracy of 81%.
The latter model was then tested in a separate cohort of 87 patients (mean age 60 years; 38% women) referred for invasive coronary angiography. Using a pretest probability of at least 15%, this model identified a group of patients with significantly higher rates of significant coronary artery stenosis, revascularization (particularly CABG), and MACE during a median follow-up of 2 years. This model performed better than the CAD Consortium clinical score, which did not stratify the cohort according to MACE risk.
Sengupta acknowledged that this is a pilot study, but said “there is a lot of promise that by using simple office-based features you can much better risk-stratify and then decide what are the next steps of your investigations that you would like to do.”
One potential use of these machine-learning models would be as a gatekeeper to decide which patients would need to undergo CT-based CAC scoring. “By developing these models, you can better risk-stratify and make more appropriate referral for coronary artery calcium scoring,” Sengupta said. “Only the people where there’s a higher likelihood of calcification being present would need a coronary artery calcium scan.”
But in remote locations where there is not wide availability of CAC scoring, “could you use this as a surrogate and use it for better defining the population and [guiding] more aggressive use of medical management?” Sengupta said. For instance, if a physician could tell a patient that—based on the machine-learning models—he or she has an 85% chance of having a CV event in the next 2 years, “that is powerful information to a patient, and then they may be more motivated to make lifestyle changes and take statins.”
Taking a broader view, Sengupta pointed out that there is a need to find ways to catch cardiovascular disease earlier in the process to improve patient outcomes. “If there is more precision in risk prediction, perhaps you can better [identify] those subsets of patients in the population that need to be tested and reduce the testing in those people that don’t need it,” he said, adding that that would reduce healthcare costs.
Doctors concerned that greater use of machine learning will put them out of work needn’t worry, Sengupta said. “It never is going to replace you. It’s only going to make you better and allow you to have your clinical judgment made more accurately.”
Todd Neale is the Associate News Editor for TCTMD and a Senior Medical Journalist. He got his start in journalism at …
Read Full BioSources
Farjo PD, Yanamala N, Kagiyama N, et al. Prediction of coronary artery calcium scoring from surface electrocardiogram in atherosclerotic cardiovascular disease: a pilot study. Eur Heart J Digit Health. 2020;1:51-61.
Disclosures
- The study was supported in part by funds from the National Science Foundation and by Heart Test Laboratories Inc, d/b/a HeartSciences. HeartSciences provided funding and signal-processed surface ECG devices.
- Sengupta reports having served as a consultant to HeartSciences, Ultromics, and Kencor Health.
- Farjo and Yanamala report no relevant conflicts of interest.
Comments