The Promise of AI for Improving Health Equity in Heart Failure
Most AI efforts in cardiology to date have focused on screening and diagnostics, but could AI be applied to closing gaps in care?
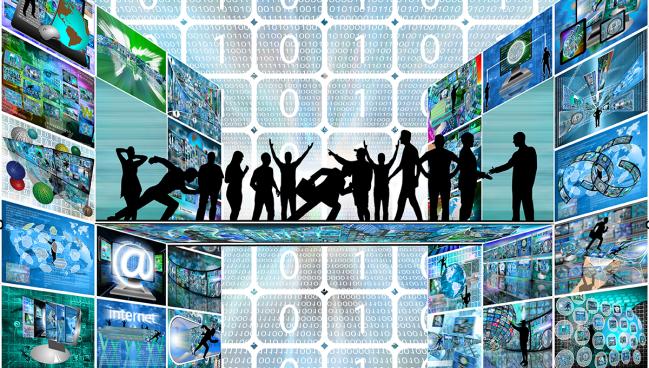
Artificial intelligence (AI) in healthcare may help physicians make sense of large amounts of data or spot patterns they wouldn’t otherwise see, perhaps allowing them to deliver higher-quality care. But it’s also possible that AI can aid in tackling inequities that persist across the healthcare system.
Disparities in medicine related to race/ethnicity, gender, disability status, sexual orientation, and other patient characteristics have long existed. In cardiology, for instance, there has been inequitable distribution of implantable cardioverter-defibrillators, cardiac resynchronization therapy, the combination of hydralazine and isosorbide dinitrate, and TAVI, Clyde Yancy, MD (Northwestern University, Feinberg School of Medicine, Chicago, IL), noted during a session on digital health last month at the Technology and Heart Failure Therapeutics (THT) 2023 meeting in Boston, MA.
And the biases underlying these disparities can infiltrate every step of the research process, from funding to dissemination of the findings, he said. That means that any models based on those data—AI or otherwise—will be similarly tainted and, if applied to clinical practice, may perpetuate the exclusion of certain patients from recent gains in the treatment of heart failure.
The field of AI is young enough that there’s an opportunity to get ahead of the issue and ensure not only that the technology is developed in such a way that it will not exacerbate existing disparities, but also that it can eventually be deployed to enhance health equity, Yancy argued.
“It’s a rare moment in time for us to be at the precipice of a brand-new technology, a brand-new way of thinking, even if it is augmented with machines, to allow us to try to unlearn some patterns of behavior over many, many decades now and relearn new ways of implementation to the extent that we can, if you will, normalize or equalize health outcomes across multiple populations, understanding that what I’m uniquely describing, then, is health equity,” Yancy told TCTMD.
I’ve never seen a scenario as it exists now for heart failure. We really have the chance to fundamentally change the outcomes. Clyde Yancy
He used his talk at THT to highlight the need for accountability: “As we rush to embrace a new technology, as we look for applications, as we use machine learning to find better ways of identifying the patients with heart failure, let’s pause for a moment and understand the extent to which unrecognized biases have not only hindered our success in implementing new strategies and deploying guidelines, but may in fact have harmed individuals.”
The idea of developing and deploying AI to reduce or even eliminate existing disparities takes on even greater importance when considering the substantial progress that has been made in the treatment of heart failure, particularly for patients with reduced ejection fraction. Yancy pointed to a recent study in JACC: Heart Failure indicating that guideline-directed quadruple therapy has shifted the mortality curves.
“I’ve never seen a scenario as it exists now for heart failure,” said Yancy, who’s been involved in the field since 1990. “We really have the chance to fundamentally change the outcomes. We haven’t cured it, but when we can take 5-year survivals of 50% out to 10-year survivals of 50%, that’s enormous.”
Data Can be Biased
Though researchers working within AI believe that the technology can be used to narrow health disparities because data are unbiased and unemotional, that’s only partly true, Yancy said. “That is correct up until the point when we understand what is the origin of the data sets upon which data aggregation and then machine learning occurs. So it is in part correct that data science almost necessarily is agnostic of the application for a given individual, but data science is not immune to having bias.”
During his THT talk, he showed a graphic summarizing the steps involved in collecting data, starting with funding, moving through motivation, project design, data collection, analysis, and interpretation, and ending with dissemination of the findings. Bias, he said, can be found at each step.
AI models incorporate these biases when they’re built using these data, and that can be addressed “with a priori awareness that this is an evident threat,” Yancy argued. “Rather than wait for us to launch any number of sophisticated algorithms that are intended to improve cardiovascular care in general, and heart failure in specific, and then as a post-hoc question try to understand if they’ve been calibrated for the different patient populations that experience heart failure, let’s say up front, a priori, these are potential areas where the genesis of the data, and thus the operationalization of the algorithms, is at risk.”
For Ashley Beecy, MD (NewYork-Presbyterian/Weill Cornell Medicine, New York, NY), who gave a talk earlier in the THT digital health session, the issue of different types of bias—computational, systemic, or human, for example—has been a focus of her institution’s AI efforts.
To mitigate this influence, Beecy’s institution has created a multidisciplinary governing body composed of legal and regulatory representatives, data scientists, implementation scientists, and informaticians who closely examine the models that have been developed. One purpose is “to understand if the population for which the model was trained is reflective of the population that the model will be implemented on,” Beecy, director of AI operations at NewYork-Presbyterian, told TCTMD.
One way bias might be uncovered is if cost was used as a surrogate for healthcare needs—leading to greater resource allocation for people with higher expenditures within the hospital system—within the data used to build the model. “What that didn’t account for was the people that didn’t have access to care, and so it perpetuated existing disparity,” Beecy said.
Because bias can be introduced at any point, what’s more important than assessing model performance is looking at the impacts over time and creating a learning health system, she said. “There’ll be influences and other biases that come later in that life cycle on how people use or adopt or implement this in their health system that could introduce other things that we would need to think about,” Beecy said.
Putting AI to Work on Health Equity
As AI technology matures and starts getting rolled out to more and more health systems, how, then, can it be used to mitigate or eliminate existing inequities in health?
Yancy pointed to an ongoing pilot project within his health system to illustrate the potential. Investigators collected information on social determinants of health among 18,000 patients, and discovered that about 11% had an overt need in one of the several domains examined. Unexpectedly, the resources required to address those needs already existed either at Northwestern or within the public health system of Cook County, which includes Chicago.
By having a more systematic way of identifying these patients, we can ensure that we try to close care gaps in an equitable way. Ashley Beecy
“If we were able to already address the explicit need that 11% of our pilot population had as the first launch of a data initiative to potentially improve outcomes, imagine what happens when we are more sophisticated with the deployment of something like a social determinants of health tool,” Yancy said. If these variables contributing to disparities in care can be incorporated into AI models as a calibration factor up front, he added, “it might make a difference.”
Beecy said AI has the potential to overcome referral bias, in which certain groups of patients don’t get the same level of care as others. One study, for example, showed that women, older patients, and individuals who were Black or Latinx who were diagnosed with heart failure after presenting to the emergency department were less likely to be admitted to the cardiology service than others.
“AI could be used to create equity in that area and flag patients for downstream testing or more advanced levels of care where it would be appropriate,” Beecy said. “By having a more systematic way of identifying these patients, we can ensure that we try to close care gaps in an equitable way.”
Partho P. Sengupta, MD (Rutgers Robert Wood Johnson Medical School, New Brunswick, NJ), whose work involves digital solutions in cardiology, agreed that AI—if developed using prospective data—can be used to address inequities. Moreover, he told TCTMD, “it should also be able to address all kinds of biases that currently exist in our modern definitions of heart diseases or how treatments are defined.”
The technology might also be used during clinical trial recruitment to ensure that the participants are representative of the heterogeneity that exists in society, he said. Then, AI can help distill the information obtained from a diverse population and provide individualized solutions. Perhaps one day physicians can target the specific components of guideline-directed medical therapy for heart failure that will most benefit individual patients.
“That’s where I think you are embracing the diversity and creating an equitable evolution of therapies,” Sengupta added.
Measured Enthusiasm
With any new technology, there’s a certain degree of circumspection needed when assessing its potential, and that’s no different for AI.
“How many different exciting initiatives have come across our radar screen only to require a lot more calibration when they finally become operationalized?” Yancy said, citing early enthusiasm for meta-analyses to streamline clinical trials and for renal denervation to provide 20- to 25-mm Hg drops in systolic blood pressure.
“Whether it’s a technique or whether it’s an intervention, early adopters almost always have unbridled enthusiasm and spectacular numbers of an effect, but that really has to stand the test of time, replication, validation of the cohorts, refinement of the technology, refinement of the intervention,” Yancy said.
AI is still at the beginning of this process, warranting some caution, “but I also see it as potentially exciting, and if we do this correctly, we can probably do something that we never thought we’d be able to do before and on a scale that we didn’t think was attainable,” Yancy said. “That is to say, with the right product in hand and the right kind of promulgation, we can probably impact the lives of many, many more patients than we ever could have before just using conventional strategies. So I do think that the posture of being circumspect was correct, but I also do think that enthusiasm for this in a measured way is also correct.”
For heart failure specifically, there’s an imperative to figure out how to get the benefits of proven, guideline-directed medical therapies to everyone who is eligible, he stressed.
“If you just think about it in the abstract academic way, artificial intelligence is for the good and we should evolve it as a discipline, but now when you think about it not only in the academic way but in the applied way to a disease process [like heart failure], this really does represent an opportunity that we’ve just simply not had before,” Yancy said.
To ensure that AI and other advanced technologies are being applied in a way that moves health equity in a positive direction, “the first step is acknowledging that this risk exists, so I’m glad that we’re having this conversation and I’m glad that Dr. Yancy brought it to the conference for further discussion,” Beecy said. After that, having multidisciplinary oversight of implementation, monitoring long-term impacts, and changing course (if needed to respond to detected bias) is key, she indicated.
For the use of AI in patients with heart failure specifically, she said, “I really look forward to seeing how it can advance the care for these patients as we start to go from prediction of disease to prognosis, and potentially to management.”
Todd Neale is the Associate News Editor for TCTMD and a Senior Medical Journalist. He got his start in journalism at …
Read Full BioDisclosures
- Beecy reports receiving consulting fees/honoraria/speaking fees from Bristol Myers Squibb and Imagen.
- Sengupta reports receiving consulting fees/honoraria/speaking fees from RCE Technologies and Echo IQ.
- Yancy reports no relevant conflicts of interest.
Comments