Machine Learning ‘Sniffs’ Out Long QT Otherwise Unseen on ECG
This tool, which mines ECG data beyond what the clinician can see, will likely ease the identification of other cardiac conditions.
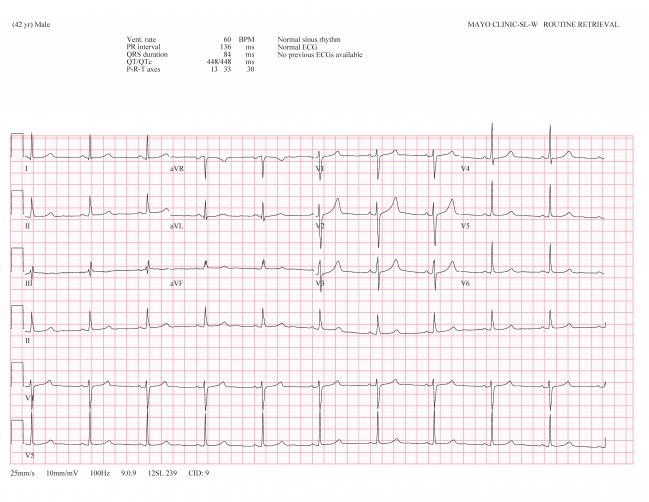
An asymptomatic male patient with definite LQTS with a normal ECG and QTc who was flagged by the AI. Photo Credit: J. Martijn Bos and Michael Ackerman.
A new artificial intelligence (AI) solution based on 12-lead electrocardiogram waveform data may be able to better identify long QT syndrome (LQTS) than what expert clinicians are able to see on their own, according to a new study.
“This is an AI-based congenital long QT ‘sniffer’ if you will,” senior author Michael Ackerman, MD, PhD (Mayo Clinic, Rochester, MN), told TCTMD. This development is “really exciting,” he said, because while it’s known that the 25-40% of people with LQTS who have normal corrected QT (QTc) values have a low risk of sudden death, “the risk isn't zero.”
LQTS is a fatal but treatable congenital condition that affects one in 2,000 people.
Their group has encountered people whose QT number is normal or close enough that their condition wouldn’t be detected by traditional ECG, he said.
“There have been tragic deaths where some of those individuals have been given QT-prolonging drugs unaware, have had other QT-aggravating diseases that seem to then be causing a more marked QT reaction than what it should have,” Ackerman explained. “So, if there is a pregenetic test way of catching these individuals, rather than waiting for these individuals to be backfilled by cascade genetic testing, then this is a wonderful solution to . . . potentially discover all long QT syndrome as soon as possible so that simple lifesaving preventative measures can be put in place.”
For the study, published online yesterday ahead of print in JAMA Cardiology, Ackerman along with lead author J. Martijn Bos, MD, PhD (Mayo Clinic), and colleagues used 12-lead ECG data from 2,059 patients who presented to their genetic heart rhythm clinic with confirmed LQTS (n = 967) or suspected LQTS (n = 1,092) between 1999 and 2018. They developed a multilayer convolutional neural network that was trained using 60% of the cohort, validated in 10%, and tested on the remaining 30%.
The neural network outperformed ECG-derived QTc classification in being able to identify patients with LQTS from those who were dismissed as normal. The same pattern was seen among the 40% of patients with a normal resting QTc (< 450 milliseconds).
LQTS Prediction by Method
|
AUC |
95% CI |
Overall |
|
|
ECG-Derived QTc Alone |
0.82 |
0.79-0.86 |
AI-ECG |
0.9 |
0.88-0.93 |
Patients With Normal Resting QTc |
|
|
ECG-Derived QTc Alone |
0.74 |
0.69-0.79 |
AI-ECG |
0.86 |
0.82-0.90 |
The “AI brain,” as Ackerman referred to the tool, improved when it was possible to analyze the mean of all available ECGs for a patient—rather than only the incidental ECG in the above results—and also was able to discriminate the three main genotypic subgroups of LQTS. “Early identification of a patient’s genetic subtype can facilitate risk stratification and disease management,” the authors write.
Universal Screening Needed
Ackerman said that LQTS is currently both under- and overdiagnosed. “We see situations where the long QT label is being prematurely and erroneously applied to patients,” which then triggers a cascade of overtreatment and overutilization of implantable cardioverter-defibrillators, he said. “But then on the other hand, we still are in a phase where we don't detect long QT families often until the first tragedy has happened.”
What’s urgently needed is a universal screening program, Ackerman continued. “It's just that we haven't had the tools to make screening a practical reality. Now, with the AI-ECG, we are getting closer and closer to having tools that makes us ready.”
I can stare at two ECGs until I'm blue in the face, but I can't pause on one over the other and say I'm a little bit suspicious here, whereas the AI brain is doing just that. Michael Ackerman
Moving forward, he said his team will need to “fine-tune the AI brain” to be able to catch all LQTS. One limitation of this study was that the neural network was only trained on patients who came in for evaluation in the first place. “That's not reflective of the general population from the standpoint of screening,” Ackerman explained. “So when you're training the AI brain to group A and group B with that setup, that's going to be different than using the AI long QT detector for a presports participation screening tool or a 6th grade or kindergarten screening tool or rooming the patient as a vital sign tool type thing.”
In an accompanying editorial, Geoffrey H. Tison, MD, MPH (University of California, San Francisco), writes: “Physicians typically interpret ECGs in a systematic manner, making it easy to forget that there is likely much more information contained in the ECG than existing interpretation techniques use. One needs only to look at the myriad of reports in recent decades that have incrementally identified novel ECG features associated with specific diseases, including for LQTS, to be reminded that there is much more information contained within ECG waveforms than we currently understand.”
“This study contributes to the important body of work demonstrating how machine learning algorithms, properly applied, can expand the utility of existing medical data sources, such as ECGs, to extract novel insights and improve performance, in many cases without significant additional invasiveness or cost,” Tison concludes. Real-world applicability of this technology, however, will “be largely dictated by the success of their integration into the clinical workflow and the scale of their adoption: key directions requiring collaboration between the investigators developing these technologies and the clinicians interpreting and deploying them in daily medical practice.”
Ackerman said he is hopeful about the future potential of the AI-ECG, even for early identification of other dangerous syndromes and conditions. Currently, he said, all patients receiving an ECG at the Mayo Clinic undergo AI evaluations for hypertrophic cardiomyopathy, atrial fibrillation, and low ejection fraction.
“I can stare at two ECGs until I'm blue in the face, but I can't pause on one over the other and say I'm a little bit suspicious here, whereas the AI brain is doing just that,” Ackerman said. “Now we're trying to figure out what is it in all of the 60,000 elements of data per ECG that the neural network is ‘seeing’ to say this person might own a mutation in their long QT code and this other person is stone cold normal.”
Yael L. Maxwell is Senior Medical Journalist for TCTMD and Section Editor of TCTMD's Fellows Forum. She served as the inaugural…
Read Full BioSources
Bos JM, Attia ZI, Albert DE, et al. Use of artificial intelligence and deep neural networks in evaluation of patients with electrocardiographically concealed long QT syndrome from the surface 12-lead electrocardiogram. JAMA Cardiol. 2021;Epub ahead of print.
Tison GH. Finding new meaning in everyday electrocardiograms—leveraging deep learning to expand our diagnostic toolkit. JAMA Cardiol. 2021;Epub ahead of print.
Disclosures
- Ackerman reports serving as a consultant for Abbott, Audentes Therapeutics, Biotronik, Boston Scientific, Daiichi Sankyo, Invitae, LQT Therapeutic, Medtronic, MyoKardia, and UpToDate.
- Bos, Ackerman, and Mayo Clinic have an equity/royalty relationship with AliveCor Inc but without remuneration thus far.
- Tison has received support from the National Institutes of Health, has previously received research grants from General Electric, Janssen Pharmaceuticals and Myokardia, and is an unpaid adviser to Cardiogram.
Comments