Normal ECG? Artificial Intelligence Disagrees, Spots Signs of A-fib
While intrigued by the technology, one expert cautions it’s hard to know “where something like this fits” in clinical care.
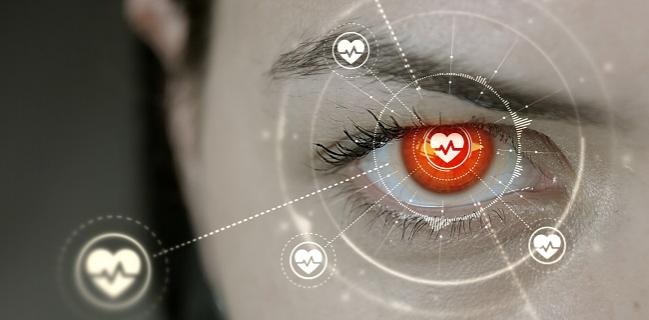
Artificial intelligence (AI) can detect signs of existing or emerging A-fib in ECGs that exhibit normal sinus rhythm, Mayo Clinic researchers have found. Their retrospective analysis, published online yesterday in the Lancet, reports a high degree of accuracy with only one ECG, and this accuracy increases when AI is applied to multiple ECGs from the same patient.
“A very common clinical scenario is that someone comes to the hospital with an ischemic stroke, and we want to know whether they have atrial fibrillation,” senior author Paul A. Friedman, MD (Mayo Clinic, Rochester, MN), noted to TCTMD. “We have done previous work using neural networks, machine learning, that found that it was extremely powerful in detecting subtle patterns [in ECG tracings], and we wondered: If someone had atrial fibrillation yesterday, is there any way that it might leave a trace of a finding on an ECG today that’s too subtle for a human to read but a computer could pick up?”
To find out, the investigators, led by Zachi I. Attia, MSc, and Peter A. Noseworthy, MD, drew upon records in the Mayo Clinic Digital Data Vault.
Friedman noted that once patients present with an ischemic stroke, it is critical to know if they have experienced A-fib so that they be considered for anticoagulation. Implantable recorders or Holter monitors are cumbersome and expensive to deploy, he said, and they miss many cases of intermittent A-fib. The idea here was to mine a standard ECG that takes 10 seconds to create.
If someone had atrial fibrillation yesterday, is there any way that it might leave a trace of a finding on an ECG today that’s too subtle for a human to read but a computer could pick up? Paul A. Friedman
From an initial data set of 1,000,000 ECGs collected at Mayo from 1993 to 2017, the researchers focused on nearly 650,000 normal sinus rhythm ECGs from more than 180,000 adults. They split the ECG records into three groups: 70% were used to train an AI system, 10% were used to validate the results of that training, and the final 20% were used to test how well AI identified A-fib on an ECG.
The AI system Mayo built is known as a “convolutional neural network,” which is a type of machine learning optimized to find patterns in visual imagery. To create it, Attia, Noseworthy, and colleagues supplied this network with an ECG representing the first recorded episode for every patient who had A-fib, as well as all ECGs for that same patient within the 31 days prior to this episode.
“We chose this window of interest with the assumption that the structural changes associated with atrial fibrillation would be present before the first recorded atrial fibrillation episode; we chose a relatively short time interval as a conservative measure to avoid using ECGs before any structural changes developed,” the researchers explain in their paper. For patients without A-fib, they supplied the neural network with their index ECG.
Using just one ECG per patient, the neural network achieved 79.0% sensitivity and 79.5% specificity for detecting A-fib. With multiple ECGs at the network’s disposal, sensitivity improved to 82.3% and specificity to 83.4%.
‘Proof of Concept’
In an editorial accompanying the paper, Jeroen M.L. Hendricks, MD (South Australian Health and Medical Research Institute, Adelaide, Australia), and Larissa Fabritz, MD (Institute of Cardiovascular Science, Birmingham, England), note that new ideas are welcome, as “silent or undetected atrial fibrillation is common and the few screening methods available are demanding in terms of time and resources.”
The current study’s hypothesis, they explain, is “that the signature of atrial fibrillation due to the structural changes in the atria could be identified by a trained network, using a standard 10-second, 12-lead ECG recorded during sinus rhythm.”
This approach could be clinically important and might “lead to a paradigm shift in recording sinus rhythm rather than atrial fibrillation on an ECG, with a specific focus on identifying structural changes. However, false negatives might also be part of the outcomes and would prevent appropriate therapy,” Hendricks and Fabritz note, adding that for this reason “the AI-enabled algorithm would require further validation in a different patient cohort, testing a healthier out-of-hospital population, as well as a rigorous prospective clinical trial assessment.”
Commenting on the study for TCTMD, Warren J. Manning, MD (Beth Israel Deaconess Medical Center, Boston), said it offers an intriguing “proof of concept” about the potential of AI for detecting A-fib from an ECG. That said, Manning seemed somewhat underwhelmed by the specifics.
“Despite a million ECGs, they only had a sensitivity/specificity of somewhere in the low 80s,” he observed. Very well-established risk factors for developing A-fib—such as a person’s age, or whether they have palpitations or consume excessive caffeine—may well have performed comparably to the neural network, Manning cautioned, concluding: “It’s hard to know where something like this fits [into] potential clinical care.”
Friedman and colleagues are moving ahead with research they hope will flesh out these initial observations.
“Once we can say if you’ve had a stroke and you’ve had atrial fibrillation yesterday, we hope to then be able to say we can treat you with a blood thinner and prevent another stroke,” Friedman said. “We’ve not done that study yet. We’ve just done the first one; [it] shows that we can find atrial fibrillation that was present and now has stopped.”
Marcus A. Banks is the 2019 recipient of the Jason Kahn Fellowship in Medical Journalism. He is currently a master’s…
Read Full BioSources
Attia ZI, Noseworthy PA, Lopez-Jimenez F, et al. An artificial intelligence-enabled ECG algorithm for the identification of patients with atrial fibrillation during sinus rhythm: a retrospective analysis of outcome prediction. Lancet. 2019;Epub ahead of print.
Hendricks JML, Fabritz L. AI can now identify atrial fibrillation through sinus rhythm. Lancet. 2019;Epub ahead of print.
Disclosures
- Friedman and Manning report no relevant conflicts of interest.
- Hendricks reports that the University of Adelaide has received on his behalf lecture or consulting fees from Medtronic and Pfizer/Bristol-Myers Squibb. He is supported by a Future Leader Fellowship from the National Heart Foundation of Australia.
- Fabritz reports receiving institutional research grants and nonfinancial support from the European Union, British Heart Foundation, UK Medical Research Council, Deutsche Forschungsgemeinschaft, and Gilead as well as being listed as inventor on two A-fib-related patents held by the University of Birmingham.
Comments