Smart Device Shows Promise in Identifying Out-of-Hospital Cardiac Arrest
Smart home devices may one day be able to recognize agonal breathing and more quickly activate the care pathway.
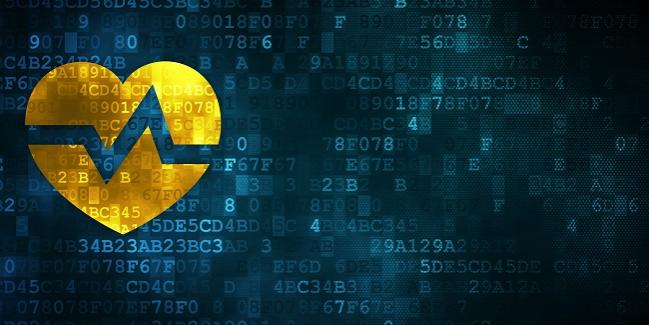
By identifying agonal breathing in a variety of bedroom-like settings, a novel machine-learning tool holds the potential to decrease the amount of time it takes for care to reach out-of-hospital cardiac arrest patients.
“The whole sort of explosion of smart devices everywhere—including smart speakers, which this is really well suited to—has enabled this totally new opportunity in so many health domains to try to identify people who are in this case having an acute emergency but also other more chronic conditions that may be worsening,” senior study author Jacob Sunshine, MD, MS (University of Washington School of Medicine, Seattle), told TCTMD. “Our goal would be to try to take advantage of this existing network of devices [and] be able to deploy it to be able to identify people who are out in the world and maybe in their homes or in hospitals or eldercare facilities to be able to have this tool available for unmonitored environments where people might experience these events.”
It may not sound like a big deal, but if you can shave a minute off of the time when someone who experiences an event gets CPR, that's actually a really big deal when you look at it from a public health standpoint. Jacob Sunshine
Using a 911 database of 162 calls with clear recordings of agonal breathing, which is caused by a brainstem reflex in patients with severe hypoxia, Sunshine, along with Justin Chan (University of Washington, Seattle), and colleagues trained a support vector machine to be able to identify this specific early marker of cardiac arrest with a specificity of 97.24% up to 20 feet away.
Their study, which was published online June 19, 2019, in npj Digital Medicine, also shows the tool’s ability to accurately identify agonal breathing in polysomnographic sleep lab recordings with concurrent snoring and obstructive sleep apnea events (0 to 0.14% false-positives) as well as in real-world sleep recordings where participants played podcasts, soundscapes, or white noise (0 to 0.22% false-positives).
Sunshine, who emphasized that the machine-learning tool is still only in the research phase, said that he envisions this technology one day being able to activate emergency medical services in order to get timely care to a patient who might not otherwise be able to call for help. “Also, you could imagine notifying a nurse or other health worker who's at an elderly care environment or a loved one who may be down the hall or even in the bed with the victim, so there's all these things where you could summon someone to get there fast,” he said. “There's still a ton of research in this area. It may not sound like a big deal, but if you can shave a minute off of the time when someone who experiences an event gets CPR, that's actually a really big deal when you look at it from a public health standpoint. If this is eventually implemented, we think it could be a reasonably cheap way to monitor people just because it takes advantage of existing devices that people already have.”
Next, the researchers are planning to strengthen their algorithm by exposing it to a wider variety of 911 call databases, potentially using international partners. “Other studies that we're considering are deploying this classifier in places that have a higher-than-average risk for experiencing these events,” Sunshine added.
Yael L. Maxwell is Senior Medical Journalist for TCTMD and Section Editor of TCTMD's Fellows Forum. She served as the inaugural…
Read Full BioSources
Chan J, Rea T, Gollakota S, Sunshine JE. Contactless cardiac arrest detection using smart devices. NPJ Digit Med. 2019;Epub ahead of print.
Disclosures
- All co-authors are inventors on a US provisional patent, submitted by the University of Washington, which is related to this work.
- Chan reports having equity stakes in Edus Health, Inc., which is not related to the technology presented in this manuscript.
Comments