In HFrEF, AI Shows Promise For Predicting Beta Blocker Response
The tool could potentially better stratify which patients will benefit and avoid adverse outcomes, authors say.
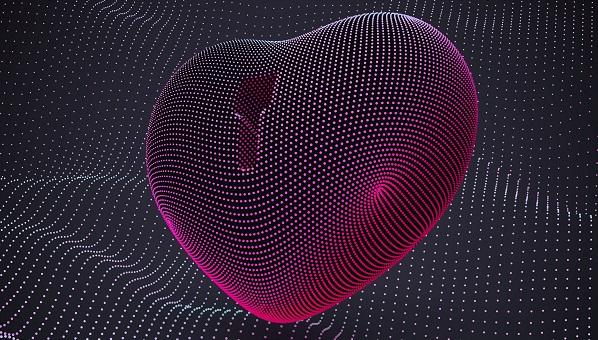
A cluster-based artificial intelligence (AI) approach has identified subgroups of patients with heart failure and reduced ejection fraction (HFrEF), both in sinus rhythm and atrial fibrillation (AF), who may or may not benefit from beta-blocker therapy.
While the tool is not able to yet pinpoint specific characteristics of patients who may be more likely to respond to these drugs—beyond what classic statistical methods might recognize—it represents a step in that direction, researchers say.
“We have developed an application of mobile machine learning that was able to define clusters of treatment response for beta blockers in patients with reduced EF,” reported Andreas Karwath, PhD (University Hospitals Birmingham NHS Trust, England), in his virtual presentation at the recent European Society of Cardiology (ESC) Congress. “The clustering is based on an objective assessment, and our robustness of validation protocols and pipeline provide reassurance of the consistency and repeatability of our findings.”
This kind of instrument “could allow for more refined stratification of treatment response to common clinical treatments, leading to avoidance of adverse outcomes,” the researchers write in a paper that was simultaneously published in the Lancet. “Prospective evaluation in a randomized setting is now warranted to evaluate if these approaches are valuable in routine practice to improve patient prognosis, particularly in conditions such as heart failure, which remains a key driver of health-care cost and poor patient quality of life.”
The “added bonus” of using the neural network-based variational autoencoders as the researchers did here is that “we can easily apply the same kind of embedding and mapping onto new data,” Karwath told TCTMD, adding that this system basically preprocesses data by reducing the number of variables while also “capturing a more complex relationship” between them.
Questioning a Cornerstone
For the study, Karwath and colleagues included 15,659 patients with HFrEF (median age 65 years; 24% women) from nine randomized controlled trials comparing beta blocker therapy and placebo with a median follow up period of 1.3 years.
As a group, patients in sinus rhythm (n = 12,822) had reduced all-cause mortality with beta blockers compared with placebo (13.9% vs 17.9%; adjusted OR 0.74; 95% CI 0.67-0.81). All but one of the six clusters of these patients identified by AI showed a mortality benefit associated with beta blockers with ORs ranging from 0.54-0.74, with the one cluster showing no effect (OR 0.86; 95% CI 0.67-1.10), made up primarily of older patients with less severe symptoms and a lower heart rate than the average.
For those with AF (n = 2,837), beta blockers did not reduce all-cause mortality compared with placebo for the group as a whole (19.7% vs 21.1%; adjusted OR 0.92; 95% CI 0.77-1.10). Four of the five clusters identified by the AI likewise showed no benefit, including one characterized by patients with low LVEF and a markedly elevated mortality risk. However, one cluster—comprised of younger patients with lower rates of prior MI but similar LVEF to the average AF patient—showed reduced mortality with beta blockers (OR 0.57; 95% CI 0.35-0.93) with a number needed to treat of 17.4.
“One of the strengths of this study is that the individual patient data used originates from a number of double-blind randomized trials,” Karwath said. One criticism might be that the trials used enrolled patients prior to the emergence of routine cardiac resynchronization therapy, neprilysin inhibitors and SGLT2 inhibitors, he conceded. “However, beta blockers still remain the cornerstone of heart failure therapy today. Finally,” he added, “the current study only focuses on mortality to avoid confounding by detection of hospitalization and other competing risks.”
His team is planning future studies in hospitalized populations to identify similar clusters of patients to see if beta blockers have the same variable effect. “In the long run, I would like to expand that onto sequences,” Karwath said, explaining that they would look at the entire care pathway for a patient and not only at a “snapshot.” This would ultimately define patient phenotypes which could then ideally be tied to patient outcomes, he explained. Multimodal studies incorporating imaging could enhance the quality of these tools even more.
This kind of decision aid could likewise be implemented for other drugs and diseases, he suggested, adding that data from a minimum of three randomized trials would be needed to do so.
Unclear Real-world Implications
In an accompanying editorial, Robert Avram, MD (University of Montreal, Canada), and Abhinav Sharma, MD (McGill University, Montreal, Canada), say the study is strengthened by using high-quality randomized controlled trial data and the objective selection of clusters. “They also did external validation using repeated assignment of clusters, across random subsets of the data and by the leave-one-trial out approach, confirming the robustness of the cluster assignment.”
The findings show the increased potential of these kinds of AI-based tools to be applied in future uses, Avram and Sharma write. “Such approaches might pave the way for personalized medicine across a spectrum of heart failure therapies and across other medical conditions.”
Still, the clinical implications remain unclear as real-world patients cannot be readily assigned to specific clusters, they say.
In discussion following the study’s presentation at ESC, session co-moderator John Cleland, MD, PhD (University of Glasgow, Scotland), said it raises numerous questions. “The biggest, of course, with any artificial intelligence presentation is: you’ve got a black box and how can we actually interrogate the analysis to identify the reasons for the findings?” he asked. “So with beta blockers I guess there’s a question of whether these differences that you’ve observed are due to differences in the efficacy of the beta blocker or differences in compliance with the beta blocker.”
One solution, he suggested, would be to use sex as a “surrogate” for testing compliance in further studies, given that women have been shown to be better compliant in prior research.
Karwath agreed that the variation autoencoder used here “doesn’t open up to see the dependencies for each of the factors influencing the output.” However, he said, the patterns underneath what is shown on the surface of each cluster’s individual characteristics “might be far more complex.”
Further, Karwath said his team did not look at compliance specifically, but acknowledged that it might be an issue; they plan to look at that more closely with future studies. “Having said that, our aim was to try and find subgroups or clusters really right from the start at baseline. And that is also demonstrated by the trial outcomes.”
Ultimately, Avram and Sharma say, “the pragmatic real-world clinical utility achieved by such algorithms will also be dictated by their successful integration into the clinical workflow. Further research on understanding the clinical utility and implementation of such artificial intelligence and machine learning-based analysis in HFrEF will be needed before such algorithms can be widely adopted and used in practice.”
Yael L. Maxwell is Senior Medical Journalist for TCTMD and Section Editor of TCTMD's Fellows Forum. She served as the inaugural…
Read Full BioSources
Karwath A, Bunting KV, Gill SK, et al. Redefining β-blocker response in heart failure patients with sinus rhythm and atrial fibrillation: a machine learning cluster analysis. Lancet. 2021;Epub ahead of print.
Avram R, Sharma A. Tailored use of β blockers using artificial intelligence. Lancet. 2021;Epub ahead of print.
Disclosures
- The study was funded by Medical Research Council, UK, and EU/EFPIA Innovative Medicines Initiative BigData@Heart.
- Karwath reports no relevant conflicts of interest.
- Avram reports receiving speaker’s fees from Servier Canada.
- Sharma reports receiving support from the Fonds de Recherche Santé Quebec and the Canadian Institute for Health Research, as well as personal fees from AstraZeneca, Novartis, Bristol Myers Squibb-Pfizer, Boston Scientific, Medtronic, Roche Diagnostics, Boehringer-Ingelheim, Novartis, and Takeda, all outside of the area of work commented on here.
Comments