To Predict Mortality After MI, Machine Learning Needs Better Intel
In order for AI-based algorithms to perform better, data sets need to become less crude, study author says.
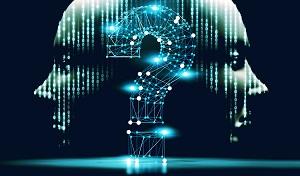
Squelching some of the mounting excitement over artificial intelligence, a new study shows no improvement in predicting in-hospital mortality after acute MI with machine learning over standard logistic regression models.
“Existing models were not perfect, and our thought was using advanced models we could derive additional insights from these presumably rich data sets,” lead author Rohan Khera, MBBS (Yale School of Medicine, New Haven, CT), told TCTMD. “But we were unable to discern any additional information, suggesting that our current way of abstracting data into fixed fields, like we do in registries, does not capture the entirety of the patient phenotype. And patients still have a lot of features that we probably capture in our day-to-day clinical care that are not put into these structured fields in a registry.”
It’s not that the data show a problem with machine learning, echoed Ann Marie Navar, MD, PhD (UT Southwestern Medical Center, Dallas, TX), who co-authored an editorial accompanying the study. “It's as much a reflection that our current statistical tools for more traditional risk prediction are actually pretty good,” she told TCTMD. “So it's kind of hard to build a better mouse trap there.”
For the study, published online this week in JAMA Cardiology, Khera and colleagues compared the predictive values of several machine-learning-based models with logistic regression for in-hospital death among 755,402 patients who were hospitalized for acute MI between 2011 and 2016 and enrolled in the American College of Cardiology Chest Pain - MI Registry. Overall in-hospital mortality was 4.4%.
Model performance, including area under the receiver operator curve (AUROC), sensitivity, and specificity, was similar for logistic regression and all machine learning-based algorithms.
Model Performance: In-Hospital Mortality Among Acute MI Patients
|
Logistic Regression |
LASSO |
Neural Network |
XGBoost |
Meta-Classifier |
AUROC |
0.878 |
0.874 |
0.874 |
0.886 |
0.886 |
Sensitivity |
0.42 |
0.43 |
0.41 |
0.44 |
0.43 |
Specificity |
0.97 |
0.97 |
0.97 |
0.97 |
0.98 |
Notably, both the XGBoost and meta-classifier models showed near-perfect calibration in independent validation, with each reclassifying 27% and 25%, respectively, of people who had been deemed low risk by logistic regression as being moderate-to-high risk, which was more consistent with observed events.
“The general conclusion that we draw is that our data streams have to become better for us to be able to leverage them completely for all clinical applications,” Khera said. “Our current data are very crude—they're manually abstracted into a fixed number of data fields—and our assumption that a model that does a little better at detecting relationships in these few variables will do better is probably not the case.”
If currently available models work, “why would you replace it with something else that has more computational power but requires more coding skill and everything involved?” Khera asked. “If both the skill set and the computational power are higher in developing such models. It only makes sense to develop such models if you're application markedly improves the rate of predictions or understanding quality or new signatures of patients.”
This means that healthcare systems have work to do, he continued. “Hospitals and healthcare systems should band together to participate in rich data-sharing platforms that can allow us to aggregate this rich information from individual hospitals into a common consortium,” Khera said, noting that current electronic health record (EHR) research is often single institution based. “What registries offer at the other end of the spectrum is you could have a thousand hospitals contributing their data.”
Similarly, he called for national cardiovascular societies “to now go to the next level by incorporating these rich signals from the EHR directly into a higher dimensional registry rather than these manually extracted registries.”
In the ‘Gray Area’
In their editorial, Navar along with Matthew M. Engelhard, MD, PhD, and Michael J. Pencina, PhD (both Duke University School of Medicine, Durham, NC), write that “when working with images, text, or time series, machine learning is almost sure to add value, whereas when working with a fewer, weakly correlated clinical variables, logistic regression is likely to do just as well. In the substantial gray area between these extremes, judgment and experimentation are required.”
This study falls in this category while also hinting at the potential benefits of machine learning. “When correctly applied, it might lead to more meaningful gains in calibration than discrimination,” they say. “This is an important finding, because the role of calibration is increasingly recognized as key for unbiased clinical decision-making, especially when threshold-based classification rules are used. The correctly applied caveat is also important; unfortunately, many developers of machine learning models treat calibration as an afterthought.”
Navar explained that the importance of calibration is dependent on how the model is being used. For example, if it is being deployed to find the patients within the top 10% highest risk in order to best dole out a targeted intervention, discrimination is more important, she said. “But if you have a model to tell somebody that their chance of a heart attack in the next few years is 20% or 10% or 15% and you're giving that actual number to a patient, you kind of want to make sure that number is as close to right as possible.” Calibration is also vital for cost-effective models, Navar added.
In this case, for risk prediction, “a traditional modeling approach is really nice because you can see what is going on with all the different variables, you can cross that to what you know about the biology and the epidemiology of whatever it is that you're looking at, and then providers can see it,” she said. “We can see how, if we're using a model, blood pressure goes up, risk goes up; someone's a smoker, risk goes up; and that's not always so obvious if you just package up a machine-learning model and just deploy it to a physician without them being able to see what's going on underneath the hood.”
For now, this advantage gives traditional models the “upper hand,” Navar said. “But that doesn't mean that the insights from those machine learning models are wrong. It just means that the other models are a little bit easier to use.”
“Recent feats of machine learning in clinical medicine have seized our collective attention, and more are sure to follow,” the editorial concludes. “As medical professionals, we should continue building familiarity with these technologies and embrace them when benefits are likely to outweigh the costs, including when working with complex data. However, we must also recognize that for many clinical prediction tasks, the simpler approach—the generalized linear model—may be all that we need.”
Yael L. Maxwell is Senior Medical Journalist for TCTMD and Section Editor of TCTMD's Fellows Forum. She served as the inaugural…
Read Full BioSources
Khera R, Haimovich J, Hurley NC, et al. Use of machine learning models to predict death after acute myocardial infarction. JAMA Cardiol. 2021;Epub ahead of print.
Engelhard MM, Navar AM, Pencina MJ. Incremental benefits of machine learning—when do we need a better mousetrap? JAMA Cardiol. 2021;Epub ahead of print.
Disclosures
- Khera and Engelhard report no relevant conflicts of interest.
- Navar reports receiving personal fees from Cerner Corporation and JAMA Cardiology outside the submitted work.
- Pencina reports receiving grants from Sanofi/Regeneron and Amgen (to Duke University) and personal fees from Boehringer Ingelheim outside the submitted work.
Comments