New Risk Models Superior to Pretest Probability at Predicting CAD
The RF-CL identified 2.5 more patients who wouldn’t benefit from more testing, but the calcium-based CACS-CL upped that to 3.5.
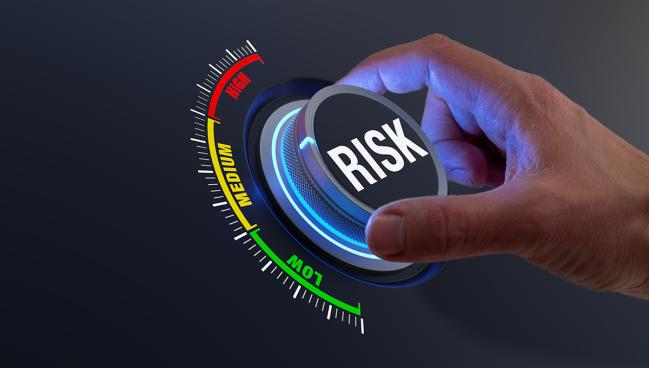
Among patients with suspected obstructive CAD, two simple models that include clinical risk factors both outperform the commonly used pretest probability (PTP) model when it comes to predicting the risk of MI and death, according to new observational data. But of the two, the model that’s informed by coronary artery calcium (CAC) scoring did best.
The risk factor-weighted clinical likelihood (RF-CL) model takes into account family history, smoking status, dyslipidemia, hypertension, and diabetes. The CAC score-weighted clinical likelihood (CACS-CL) algorithm goes a step further by incorporating calcium into the risk-factor model.
Compared with the standard PTP model, which includes only age, sex, and type of symptoms, the RF-CL and the CACS-CL identified 2.5 and 3.5 times more patients, respectively, who didn’t stand to benefit from further diagnostic testing. Both of the newer models were introduced in 2020, as reported by TCTMD.
Prior studies have shown more-potent predictive power with adding CAC to standard risk assessment, but it remains unclear whether patients for whom further tests are deemed unnecessary do indeed remain free of cardiovascular events.
“These two models have the advantage that they are very accurately calibrated to the prevalence of obstructive disease in these patients,” explained lead author Simon Winther, MD, PhD (Gødstrup Hospital, Herning, Denmark), to TCTMD. “Despite that we are down-classifying a lot of patients, the patients which are down-classified for not testing have an excellent prognosis. Our conclusion is that this seems safe, but of course we need to test this in a randomized setup. But this is very encouraging for the models and [their] application in the future.”
Similarly, Omar Dzaye, MD, PhD (Johns Hopkins University School of Medicine, Baltimore, MD), who commented on the findings for TCTMD, said the new models “are great in terms of combining data with risk factors like family history for coronary artery disease, dyslipidemia, hypertension, diabetes, and smoking, and if they now include also coronary artery calcium, they definitely exhibit better predictive accuracy than models based only on age, sex, and symptoms like the pretest probability test.”
Further, he said, the findings support relying on the so-called “power of zero” in predicting low risk for patients with a zero CAC score.
Outcomes in Two Cohorts
For the study, published in the November 22, 2022, issue of the Journal of the American College of Cardiology, Winther and colleagues applied their models as well as the PTP to two cohorts from the Western Denmark Heart Registry (n = 42,784) and the randomized PROMISE study (n = 3,952). The cohorts included patients without previously diagnosed coronary disease presenting with symptoms suggestive of obstructive CAD who were referred for noninvasive testing.
Both the RF-CL and CACS-CL models down-classified 45.2% and 60.2% of patients to a very low likelihood (≤ 5%) of CAD, which was substantially more than the 18.0% down-classified with the PTP. Also, the CACS-CL model categorized more patients as having a high likelihood of CAD (> 50%) compared with both the PTP and RF-CL (2.7% vs 0.9% and 0%, respectively).
Over a median follow-up period of 3.6 years, the rates of nonfatal MI and death were 1.2% and 2.6%, respectively. Despite the high amount of reclassification done by the models, all three resulted in low annualized event rates of nonfatal MI and death (RF-CL 0.51%, CACS-CL 0.48%, and PTP 0.37%).
Both the RF-CL (C statistic 0.64; 95% CI: 0.63-0.65) and CACS-CL (0.69; 95% CI 0.67-0.70) were deemed superior to the PTP (0.61; 95% CI 0.60-0.62).
One of the main advantages of these models are that they can be used to assess risk using a table, as opposed to other models that require input of information into a computer calculator, Winther said. “[They are] very clinically adaptable,” he said. “You can just look up a table and a graph and get the estimates. This is a positive thing.”
He would like to see these new models included in future guidelines, but acknowledged that randomized trials will be necessary before that can happen.
Role of CAC?
In an accompanying editorial, Khurram Nasir, MD, and Safi U. Khan, MD (both Houston Methodist DeBakey Heart & Vascular Center, TX), say the study’s message that using the RF-CL model without further upstream CAC testing after a patient has been reclassified as low risk “seems reasonable.” Still, they point out several nuances in the study’s methods that they argue demonstrate the predictive power of CAC, especially when it is zero.
“Although atherosclerosis presence and severity track with traditional cardiovascular risk factor burden, it is widely documented that there is a mismatch between markers of predicted and actual disease in both symptomatic and asymptomatic patients,” Nasir and Khan write. “CAC is a measure of disease rather than underlying risk, and that is why disease-based models are so much more accurate. Although the RF-CL strategy appears promising at first glance, we believe it may be preferable to use a low-cost, low-radiation, and widely accessible CAC test to provide better diagnostic and prognostic value.”
They acknowledge the lingering resistance in the cardiology community to adopting CAC testing and attribute some of this to the lack of a “more strongly” worded endorsement of CAC testing in the most-recent chest pain guidelines.
Nasir and Khan laud the authors for their contribution toward “dispelling existing clouds of uncertainty with this pragmatic approach.” And they fully support additional clinical trials “to clarify the impact of these promising strategies vs current standard of care approaches not only for identifying the right patients for advanced imaging, but also for determining how these strategies will affect subsequent patient outcomes,” the editorialists write.
Still, they conclude, “we also cannot afford to overlook the potential value of the information we already possess. Until then, let us take a moment to agree on how to develop best practices on the basis of what we now know to minimize our ongoing losses and maximize future gains.”
Yael L. Maxwell is Senior Medical Journalist for TCTMD and Section Editor of TCTMD's Fellows Forum. She served as the inaugural…
Read Full BioSources
Winther S, Schmidt SE, Foldyna B, et al. Coronary calcium scoring improves risk prediction in patients with suspected obstructive coronary artery disease. J Am Coll Cardiol. 2022;80:1965-1977.
Nasir K, Khan SU. Power of zero as gatekeeper for stable chest pain patients minimizing losses and maximizing gains. J Am Coll Cardiol. 2022;80:1978-1980.
Disclosures
- The PROMISE study was supported by grants from the National Heart, Lung, and Blood Institute.
- Winther reports receiving support from the Novo Nordisk Foundation Clinical Emerging Investigator grant.
- Nasir reports serving on the advisory board of Novo Nordisk, Novartis, and Esperion; serving on the speakers bureau of Amgen; and receiving partial support for research from grants from the National Institutes of Health, Novartis, Esperion, and the Jerold B. Katz Academy of Translational Research.
- Khan and Dzaye report no relevant conflicts of interest.
Comments