AI-Enabled Algorithm Succeeds in Identifying Diabetes From ECG Data
The DiaBeats tool needs to be externally validated before clinical use, but it holds potential, Rickey Carter says.
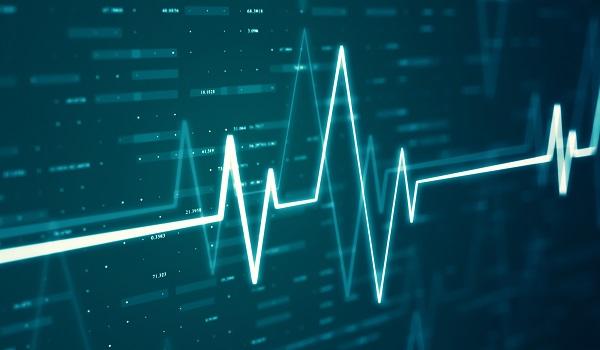
A new machine-learning tool can identify diabetes and prediabetes using ECG data, according to research recently published online in BMJ Innovations.
Similar artificial intelligence (AI) tools have been developed to quantify coronary calcium, detect long QT syndrome and new-onset atrial fibrillation (AF), and estimate physiologic age based on ECG data, but this study is one of the first to seek to use tracings to identify diabetes, which is notoriously underdiagnosed.
“The motivation for the study was to search for noninvasive and inexpensive ways to pluck the disease in its early stages,” senior author Hemant Kulkarni, MD (Lata Medical Research Foundation, Nagpur, India), told TCTMD in an email. “Cardiac autonomic perturbances occur early during the diabetic process even in the absence of dysglycemia. Therefore, we decided to use ECG as a detection strategy and combine it with the power of AI/[machine learning] to detect diabetes and prediabetes.”
Because the DiaBeats algorithm hasn’t yet been externally validated, Kulkarni called their research “proof-of-concept.” However, its potential implications for the future of diabetes screening are important, “especially in low-resource settings,” he added.
Training, Testing the Algorithm
For the study, Anoop Kulkarni, PhD (Lata Medical Research Foundation), Kulkarni, and colleagues included clinical data from 1,262 individuals of the Sindhi population from central India (mean age 48 years; 61% women). The total 10,461 time-aligned, digitally recorded heartbeats were split into training, validating, and independent testing sets.
The overall prevalence of type 2 diabetes and prediabetes were 31.7% and 13.4%, respectively. In the independent test set, the algorithm predicted whether the individual belonged in the no diabetes, diabetes, or prediabetes cohorts with 97.1% precision, 96.2% recall, 96.8% accuracy, and 96.6% F1 score with low calibration error (0.06).
“The limitations of oral glucose tolerance test and HbA1c in population screening for diabetes are well known,” the authors write. “In comparison, there are clear benefits in using ECG as a gatekeeper for diabetes/prediabetes,” namely that it is noninvasive, relatively inexpensive, and suited to telehealth, they add. Also, “an ECG can yield a far richer information spectrum about coexisting cardiovascular conditions, and therefore, simultaneously act as a first-level screen for the overall cardiovascular health as well.”
Kulkarni said their next step will “be to establish the robustness of this algorithm in a variety of settings. Once that happens, it would also be important to establish that the features being detected are specific to diabetes as several comorbidities (for example, hypertension, obesity, dyslipidemia) tend to aggregate with diabetes.”
Their next phase of research will involve the development of strategies to screen for diabetes using wearable devices that record ECG, he added.
‘Distal Biomarker’
“When I think of diabetes, you have period of hyperglycemia, hypoglycemia [and] there's treatment, there's a lot of things going into managing the glucose profile, so it's so much fundamentally different from looking at something like potassium levels,” he said. There might be truth to the suggestion of some cardiac remodeling happening as a result of hyperglycemia, but the question remains unanswered as to whether or not that is pronounced enough to be detected on ECG, Carter continued.
Diabetes is well known as a “poster child” of the type of disease that is hard to identify from purely electronic health record data, he explained. “The ECG is a little different, but at the same time, it's hard to imagine such a high level of performance with just an ECG alone.”
Carter said he could see future potential for this type of algorithm once it’s externally validated, but that its specificity might be lacking compared to a tool seeking to identify something like AF, for example. “If [the ECG algorithm says]: ‘Well, it looks like you might have diabetes,’ people may want some certainty that it truly is diabetes and not some other conditions. Whereas with A-fib, the arrhythmia would be very characteristic of A-fib.”
Ultimately, this paper is a good example of using AI to find a “distal biomarker—it’s an initial measured value that looks promising and the initial test and development creates a lot of interest and excitement, but it takes time to realize the benefits of that,” Carter explained. “That's going to be this translation part.”
Yael L. Maxwell is Senior Medical Journalist for TCTMD and Section Editor of TCTMD's Fellows Forum. She served as the inaugural…
Read Full BioSources
Kulkarni AR, Patel AA, Pipal KV, et al. Machine-learning algorithm to non-invasively detect diabetes and pre-diabetes from electrocardiogram. _BMJ Innov_. 2022;Epub ahead of print.
Disclosures
- This study was funded by Lata Medical Research Foundation’s internal funding mechanism.
- The authors report no relevant conflicts of interest.
Comments