Deep-Learning Tool Can Gauge Plaque Burden, Stenosis Severity on CCTA
The algorithm shows promise for predicting future events and could potentially be integrated into on-site plaque analysis.
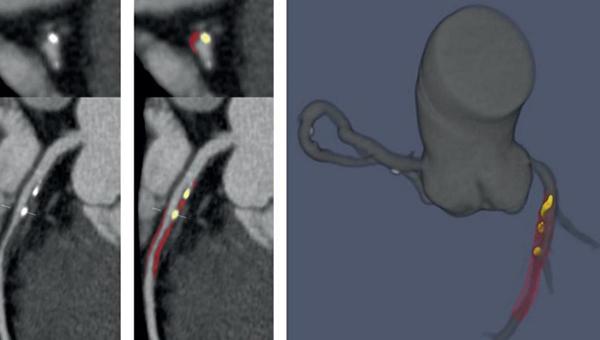
LEFT: Deep learning-enabled CCTA showing noncalcified (red) and calcified plaque (yellow). RIGHT: 3D rendering of coronary tree showing deep learning-based plaque segmentation (Photo Credit: Damini Dey)
An artificial intelligence (AI)-based tool can rapidly and accurately measure plaque volume and stenosis severity from coronary CT angiography (CCTA) images in patients with stable chest pain, according to new data. It also has the potential to better predict future MIs.
Measuring coronary plaque, a painstaking and effortful task for even the most trained physician, is not performed for every patient who presents with stable chest pain, but rather stenoses are assessed visually. In some cases, private companies can perform off-site plaque analysis, though this process can be cumbersome and expensive.
With this new algorithm, “plaque assessment could be done simply, rapidly, and efficiently on site using this AI-enabled tool,” senior author Damini Dey, PhD (Cedars-Sinai Medical Center, Los Angeles, CA), told TCTMD. Moreover, the additional prognostication analyses she and her colleagues performed using SCOT-HEART patient data demonstrate that the tool gives “enhanced cardiac risk prediction.”
This kind of deep-learning system “has the potential for integration into routine CCTA workflow, where it could function as a second reader and clinical decision support tool,” the authors write. “By providing automated and objective results, deep learning could reduce interobserver variability and interpretative error among physicians. The system could also be used to prescreen CCTA scans, flagging patients with obstructive disease who should be prioritized for reporting.”
Commenting on the study for TCTMD, Todd Villines, MD (University of Virginia, Charlottesville), said the technology has the potential to shift the way CCTA can be of use both diagnostically and prognostically. “Currently, coronary CTA studies are interpreted much like an invasive coronary angiogram that overly focuses on lumen diameter stenosis,” he explained. “This type of analysis harnesses the true power of noninvasive coronary imaging with contemporary coronary CTA and will further inform the use of preventive therapies at the patient level and allow for serial CTA studies to serve as an imaging surrogate for pharmaceutical intervention trials.”
Faster, Accurate Interpretation
For the study, published this week in the Lancet: Digital Health, Andrew Lin, MBBS (Cedars-Sinai Medical Center, Los Angeles, CA), Dey, and colleagues first trained a novel deep-learning convolutional neural network to segment coronary plaque on data from 921 international patients (5,045 lesions) who underwent CCTA between November 2010 and January 2019 for a range of CAD presentations, including stable angina, acute MI, and post-MI. Advanced imaging cardiologists also reviewed and analyzed each patient’s scans for comparison.
The deep-learning tool took a mean 5.65 seconds to analyze each patient’s plaque, which was substantially faster than the mean 25.66 minutes it took for the human experts. Overall, the algorithm was in excellent and good agreement, respectively, with expert reader measurements for total plaque volume (intraclass correlation coefficient [ICC] 0.964) and percent diameter stenosis (ICC 0.879; both P < 0.0001). Compared with IVUS, which was performed in external validation cohorts of 175 and 50 patients, the deep-learning tool showed excellent agreement for both total plaque volume (ICC 0.949) and minimal luminal area (ICC 0.904).
To evaluate the prognostic value of their validated AI tool, researchers then looked at its ability to predict subsequent events from the baseline scans of 1,611 patients from the SCOT-HEART trial, 2.5% of whom reported MI over a median follow-up period of 4.7 years. The deep-learning tool showed that a total plaque volume of 238.5 mm³ or higher was associated with a fivefold increased risk of MI (HR 5.36; 95% CI 1.70-16.86) after adjustment for the presence of deep learning-based obstructive stenosis (HR 2.49; 95% CI 1.07-5.50; P = 0.0089) and the ASSIGN clinical risk score (HR 1.01; 95% CI 0.99-1.04; P = 0.35).
The authors argue that while “visual estimation of luminal stenosis remains the cornerstone of CCTA reporting,” reader experience and training might vary, and this could interfere with the ability to reliably grade stenosis.
An AI-based system could also be useful in cases when disagreement over the presence or absence of obstructive CAD occurs, they add, noting that this is “common even among experienced expert readers.”
Finally, the authors say that this tool might also be useful for “excluding severe obstructive disease and reducing unnecessary invasive angiography.”
The real potential for this kind of automation lies in the ability to use a standard desktop computer, according to Lin and colleagues. “Although quantitative plaque analysis remains investigational, continued advancements in hardware (eg, graphics processing units) and software (eg, cloud-based computing services) will improve the time and computational efficiency of our deep-learning system, thus increasing its potential for future clinical application,” they write.
While no cost-effectiveness analyses have yet been done, Dey said she predicts this tool will save money, as well as time, by being a “workstation-based solution” that could operate within the CT department. “We could do this on-site very quickly for all the patients of the day or selected patients of the day, whatever is the easiest,” she said.
The next step will be to conduct further validation, especially in prospective clinical data, Dey said. “Then [we’ll try] to get it ready to put it into the hands of the physicians.”
Yael L. Maxwell is Senior Medical Journalist for TCTMD and Section Editor of TCTMD's Fellows Forum. She served as the inaugural…
Read Full BioSources
Lin A, Manral N, McElhinney P, et al. Deep learning-enabled coronary CT angiography for plaque and stenosis quantification and cardiac risk prediction: an international multicentre study. Lancet Digit Health. 2022;4:e256-65.
Disclosures
- Lin reports no relevant conflicts of interest.
- Dey reports receiving software royalties from Cedars-Sinai Medical Center outside of the current work and holding a single patent.
Comments