ECG Images, Thanks to AI, Can Be Used to Detect Low LVEF
The model also is able to predict which patients who don’t yet have an LVEF < 40% will go on to develop systolic dysfunction.
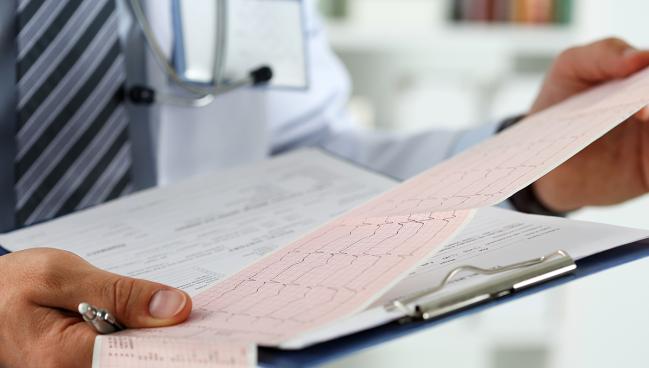
Through a deep-learning model, researchers have shown that ordinary ECG images can be leveraged to identify patients with an LV ejection fraction below 40%. Their approach also appears able to pinpoint individuals who don’t yet have LV systolic dysfunction but are at increased risk of developing it in the future.
The results, which applied artificial intelligence (AI) to nearly 400,000 12-lead ECGs and corresponding echocardiographic data from the same patients, were released online last week ahead of print in Circulation.
It’s not the first time that AI has been applied to ECGs in the hopes of mining valuable information. As reported by TCTMD, prior studies have used raw signal data from ECGs to detect LV systolic dysfunction. Other applications have included long QT syndrome, diabetes, atrial fibrillation, coronary calcium, and even “physiologic age.”
Anyone can upload a photo of an ECG to try out the tool used in the current study, which the investigators have made available through Yale’s Cardiovascular Data Science (CarDS) Lab website. Rohan Khera, MD (Yale University, New Haven, CT), senior author of the new paper, said that what stands out about their approach is that it uses ECG images, not raw signals. The challenge with the latter is that clinicians don’t have easy access to the data.
“A doctor sitting with a patient has no way to use this mechanistic information,” he told TCTMD. “What we’re working on now is very practical. . . . You can take a photo of the ECG, and it can give you a prediction on whether someone has low LVEF or not. Every ECG that’s done in any health setting, or has been done historically, you can go back and pull them and run [the model].”
The clinical need is great: asymptomatic patients with an LVEF < 40% have an eightfold higher risk of heart failure and double the risk of premature death, the paper notes.
“[Asymptomatic] LV systolic dysfunction is treatable, and it’s considered stage B heart failure. . . . Guidelines recommend that you should treat these patients with guideline-directed medical therapy: beta-blockers, ACE inhibitors, and so forth,” said Khera. “The challenge has been there hasn’t been a test that’s really suitable for [use in] mass screening.”
You can take a photo of the ECG, and it can give you a prediction on whether someone has low LVEF or not. Rohan Khera
Led by Veer Sangha, BS, and Arash A. Nargesi, MD (both from Yale University), the researchers used 12-lead ECG signal waveforms collected at Yale New Haven Hospital from 116,210 individuals between 2015 and 2021, pairing them with each patient’s most recent transthoracic echocardiogram (TTE) of the last 15 days.
The resulting model had a sensitivity of 0.89, specificity of 0.77, positive predictive value of 0.26, and negative predictive value of 0.99 for detecting LVEF < 40% on TTE. Patients with an ECG that suggested LV systolic dysfunction had much higher odds of having their TTE show the same finding (OR 27.5; 95% CI 22.3-33.9).
Of the patients who initially had a false positive—meaning that the ECG images suggested reduced LV function but the TTE did not—23.5% developed LVEF < 40% over a median follow-up of 3.2 years. Of those who initially had a true negative—neither the ECG image nor the TTE suggested reduced LV function—6.8% developed LVEF < 40% during that time frame. Thus, having a positive result on ECG in the absence of LV systolic dysfunction at the time of screening was associated with nearly quadruple the risk of a patient eventually developing it (HR 3.9; 95% CI 3.3-4.7). Even when taking into account the person’s age, sex, and baseline LVEF, having a positive screen approximately doubled the risk of future LV systolic dysfunction (adjusted HR 2.3; 95% CI 1.9-2.8).
“We’re definitely picking up on some subclinical substrate of future disease based on that baseline ECG, which is really remarkable. It’s a new digital marker for future LV dysfunction, and it’s a very robust one,” said Khera.
Also important, he added, is that the model can be applied independently and locally at different institutions. It was validated in outpatient Yale New Haven Hospital clinics and externally through ECG images taken at Cedars-Sinai Medical Center (Los Angeles, CA), Lake Regional Hospital (Osage Beach, MO), Memorial Hermann Southeast Hospital (Houston, TX), and Methodist Cardiology Clinic (San Antonio, TX), as well as through the prospective Brazilian Longitudinal Study of Adult Health.
Discrimination power was high across various ECG image formats and calibrations. In the internal validation, it had an area under the receiver operating characteristic curve (AUROC) of 0.91 and an area under the precision-recall curve (AUPRC) of 0.55. Across the other settings, the AUROC ranged from 0.90 to 0.95 and the AUPRC from 0.45 to 0.88. The most important areas for prediction of LV systolic dysfunction were those corresponding to the anteroseptal and anterior leads.
“This approach represents an automated and accessible screening strategy for LV systolic dysfunction, particularly in low-resource settings,” the authors conclude. They highlight the fact that “the algorithm was developed and tested in a diverse population with high performance in subgroups of age, sex, and race, and across geographically dispersed academic and community health systems.”
At this point, the model is still investigational and not yet approved by the US Food and Drug Administration, Khera noted. Their hope is to develop AI-based ECG screening approaches for a variety of cardiovascular conditions, with the aim of helping clinicians know when patients need to undergo additional testing, he said. Just last month, the Yale team reported details in npj Digital Medicine on how single-lead ECGs from wearable devices might prove useful.
Caitlin E. Cox is News Editor of TCTMD and Associate Director, Editorial Content at the Cardiovascular Research Foundation. She produces the…
Read Full BioSources
Sangha V, Nargesi AA, Dhingra LS, et al. Detection of left ventricular systolic dysfunction from electrocardiographic images. Circulation. 2023;Epub ahead of print.
Disclosures
- Sangha reports no relevant conflicts of interest.
- Khera reports being a co-inventor of US provisional patent applications 63/177,117; 63/428,569; and 63/484,426, and being a founder of Evidence2Health, a precision health platform to improve evidence-based cardiovascular care.
Comments